Traditional relational data won’t cut it. AI demands flexible data schemas, so AI models get the information they need, argues Michael Cohen, global chief data and analytics officer at Plus Company.
Michael Cohen specializes in transforming businesses with data-driven management systems. As global chief data and analytics officer at Plus Company, a global network of creative agencies that offers marketing services and software, Cohen led the creation of a system called AIOS that offers a comprehensive view of the customer journey that makes real-time recommendations to optimize marketing performance.
A former member of the marketing faculty at the New York University Stern School of Business, Cohen argues that the successful use of AI demands a paradigm shift in how organizations store, organize, process, and think about their data. The relational databases that have dominated IT for decades are not ready to handle the kinds of questions that can make AI applications so valuable and innovative.
The work required is clearly challenging – but the steps CIOs who want to guide their enterprises to take advantage of AI are clear, according to Cohen. He sat down with Robert L. Scheier to explain how CIOs should adapt. This interview has been edited for length and clarity.
Robert L. Scheier: When you talk about a major shift in managing data, what exactly are the changes businesses need to make, and why is it important?
Michael Cohen: To store their data with more flexible and retrofittable data schemas – which describe how the various data elements, such as customer name and address are related -- in much more AI-useful ways so models have the data they need to deliver the best results.
This is a shift from the traditional approach. The de facto standard for knowledge management is to get data into relational databases, where data is stored with a standard set of schemas – this row is for the customer’s first name, this row is their last name. The analytic tools we’ve used for the last 30 or 40 years can then sort the rows and columns to make correlations and tell you about business results or trends. But you are limited by what you can ask by how you set up the initial schema.
What about AI makes the traditional approach to data unsuitable?
A service agent (human or AI) might ask a question that requires access to multiple types of data. Where the customer is calling from, for example, requires geo-spatial data. Identifying their accent or other cultural markers in their voice requires audio data. How often they called about the same issue may require text or data from a customer relationship management system. All this data must be available both for training and for answering queries in real time. If that data isn’t available, or if the schema isn’t flexible or general enough to find and provide this data, the rep or AI service agent can’t give the best response. That means a frustrated customer and higher call-handling costs.
A model might also need different and segregated information from the cloud, a desktop, and the devices at the edge - the Internet of Things. This is very different from traditional hard-coded queries, which say something like “sort this list, or do these pivots on a table of predefined information.”
Now multiply that by all the unpredictable questions your employees might need to ask an AI model, and the cost implications if you haven’t stored your data properly for AI to use. You also need governance to determine which AIs to use for which problems to achieve the highest performance at the lowest cost.
So, what’s an ideal data architecture for AI applications?
First, implement a data organization and principles in which each database is managed locally – maybe by a specific server, device, or business unit -- but according to central rules and standards. Those common rules and standards make it easier for all your data to be easily accessed by the AI model.
Second, your data architecture must federate all types of data – structured, semi-structured and unstructured – as well as various modes such as voice, video and images as well as text. You will also need a way to relate the various data elements, known in AI as tokens, to get the best results. The major cloud providers and leading cloud data storage vendors have or will provide solutions that allow users to build such systems using relatively easy “no code/low code” kits.
This sounds expensive.
Not necessarily. First, if you’re using a SaaS platform the vendor is holding your data and is almost certainly working to make it available for use with AI in a well-governed way. Many cloud providers offer open source as well as paid solutions with varying implementation costs depending on the complexity and novelty of the models used. Costs will continue to drop as most AI use cases don’t require the biggest, most expensive models, and their costs will also keep dropping.
Much depends on the needs of your business and your internal skills. You might be fine using the AI capabilities Salesforce is building into its SaaS products. If you have unusual needs and staff savvy with using open-source AI tools, you can build purpose-built applications that can deliver substantial savings.
Take the example of producing an advertisement. Traditionally a production team assembles the images or videos, and the ad copy, in various forms for placement in specific media. This is slow and labor intensive and produces only a few variations of the ad. An experienced advertising professional could prompt an application development AI to write the code, and set up the supporting IT infrastructure, for an application that produces virtually endless variations of the ad. It could also categorize the ads and their components to track their performance and reuse their elements. This could dramatically cut labor costs, speed turnaround and reduce friction between historically separate creative and media teams.
What approach is available for businesses that want or need to keep their data in-house?
It depends on what you’re utilizing today. AI platforms from vendors such as Databricks and AWS are delivering new features to make your stored data more AI-friendly. If you’re developing more of your systems in-house, you need to ask yourself whether it’s time to migrate to the cloud or which sort of hybrid model you could institute to get started.
Depending on the size of your company, it might cost $100,000 to bring an enterprise level consultant to assess the situation and make business-oriented suggestions. This includes describing the timing and size of the value to the business, along with the key performance indicators to measure its success.
You could compare suggestions from a few consultants and triangulate their findings before deciding which data management project to take on and building a business case for it. The actual data management improvements could cost several million dollars for a business which, if you’ve done your business case right, should demonstrate value to the business in a short amount of time.
What ROI story can a CIO tell their board about this investment?
There are huge opportunities for AI to reshape employee roles and responsibilities to cut costs and cycle time everywhere from finance to sales and marketing. By making it easier for an employee to get information using everyday language and for an AI agent to act on it, individuals can oversee more types of tasks.
Take advertising. Historically, you needed one team to develop the big idea, the creative, then another to build the content, then a team to customize it and deliver it through channels such as email, television ads or Web ads and social media. Now one person can do it all, from developing an idea to using AI to adapt it for a campaign for TikTok, Google, Facebook. AI can also use information about how the ads performed to iteratively fine tune the content and its delivery for anything, from the visuals to the messaging to the pricing.
What steps should a CIO take first to adapt to this new environment?
Start experimenting with very specific subsets of your data that pose relatively lower risk. Then as you learn how these new data management techniques work you can adopt them in higher-stakes parts of the business. But keep your options open because the technology is changing quickly, as is the regulatory environment. It’s difficult to predict which technologies and which vendor will leapfrog another in either capability, or which role less expensive, open-source AI will play. With a new administration and Congress, I expect a less restrictive regulatory environment that makes experimenting with certain models easier but not without uncertainty about how end users and customers in the market will respond to their output.
Get some outside help from a partner who can draw on their experience with other clients to help evaluate which technologies are right for your business. Educate yourself about what capabilities the various AI vendors offer to meet your needs at any time. Keep your board and business partners informed and be ready to pivot quickly as the vendors’ capabilities and your needs change.
Finally, find the people on your team who tend to want to experiment, to use new tools. They are the ones who will figure out how to use AI in a new way. But remember the quality of the results is directly relative to AIs access to the context relevant data.
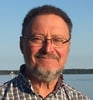
Written by Bob Scheier
Bob Scheier is a veteran IT trade journalist and IT marketing writer.